Responsible AI: A step toward inclusive, ethical and responsible use of artificial intelligence
- Dr Bidit Dey
- Apr 15
- 8 min read
Executive Summary: Artificial Intelligence (AI) holds transformative potential that could significantly impact the lives of billions worldwide. However, the design and deployment of AI have raised significant concerns due to inherent limitations. The prevalence of bias and discrimination in AI systems can result in outcomes that exacerbate existing societal issues. Gender and race-related biases, in particular, become evident, as AI is often trained on historical data that reflects skewed representations. Consequently, various sectors have faced detrimental effects from AI-driven decisions, undermining the efficacy of this technology. Nonetheless, AI is rapidly evolving and increasingly integrating into different aspects of personal and public life. Rather than stifling its potential, it is imperative to develop innovations, designs, and applications within a regulatory framework that ensures a responsible and equitable use of AI—one that benefits the broader population and contributes to the ongoing fight against discrimination and social exclusion.
This article explores how AI can introduce biases and examines the measures that various governments and private sector organizations are implementing to mitigate these risks and challenges.
Introduction:
Artificial intelligence is becoming a vital force in driving economic growth, showcasing its ability to transform business operations and improve the quality of life for millions worldwide. In light of this potential, governments worldwide are actively promoting the accelerated adoption of AI. However, this surge in adoption brings with it a series of challenges and risks that necessitate careful oversight. Ensuring the safe and responsible development and use of AI is of utmost importance, serving as a central theme for both government policies and private sector strategies.
Responsible AI is defined as a framework for the development and deployment of AI systems that prioritize ethical principles, societal well-being, and human oversight, ensuring that AI benefits society while minimizing risks. There is an increasing imperative for the implementation of responsible AI, particularly given the inherent limitations of AI that can result in biased, unethical, and discriminatory outcomes.
Why and how AI can have biases and exclusion
Artificial intelligence systems often exhibit a concerning lack of inclusivity, primarily due to their reliance on biased datasets that reflect the societal inequalities they aim to address. This dependence results in discriminatory outcomes that can further marginalize already vulnerable groups. The development of AI models typically involves reflecting on extensive datasets, which can often be skewed. As such, AI can potentially fail to capture human diversity, which is characterized by differences in race, gender, and other factors. Consequently, the outputs generated by these AI systems are not only susceptible to biases but can also inadvertently perpetuate existing exclusion and discrimination within a society.
AI algorithms can produce unfair or discriminatory outcomes, even when using diverse datasets. Many historical datasets do not adequately capture the characteristics of various communities, often neglecting vital perspectives that could enrich the AI's understanding. Furthermore, AI outcomes can be influenced by discriminatory perspectives and narratives that are used and propagated across social media and other digital platforms. For instance, research shows that women leaders are more likely to receive abuse on X (formerly known as Twitter) than male leaders[1]. Figure-1 shows the summary of the sentiment analysis.
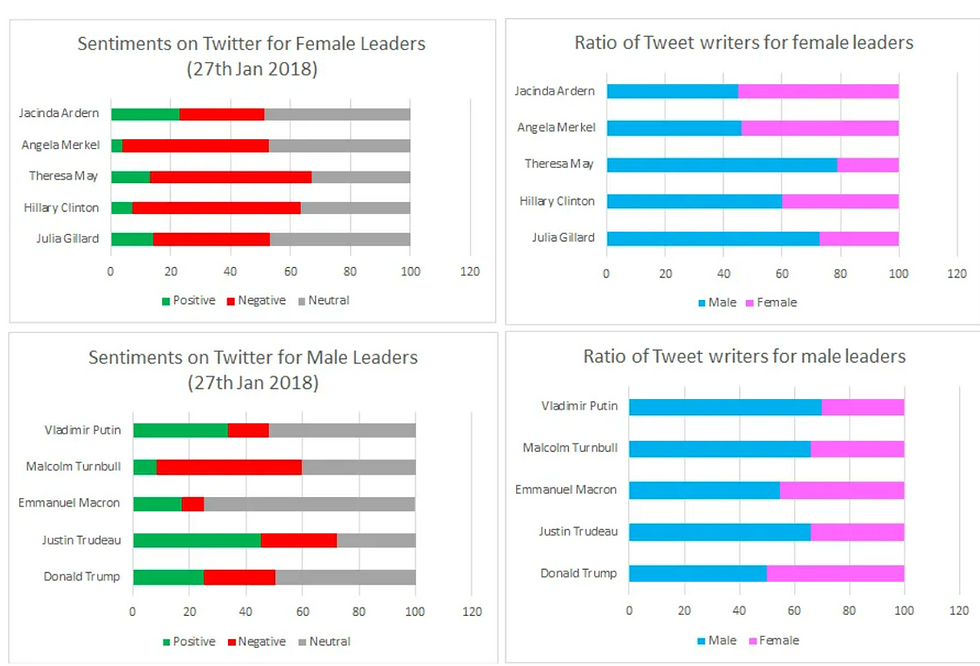
Decisions related to job interviews, medical procedures, and loan approvals are increasingly influenced by machine learning (ML) algorithms. The rising investments in ML algorithms are associated with improvements in business efficiency, often surpassing 30%[1]. The implementation of ML models and the management of individual data presents significant risks, particularly ethical challenges that cannot be ignored. Industry experts are emphasizing the critical issues surrounding bias and discrimination within AI systems, which must be addressed to ensure fair outcomes.
If developers working on these technologies lack diversity or have a limited understanding of its various manifestations, there is a significant risk of overlooking or inadequately addressing the biases inherent in the data or algorithms. Such intrinsic challenges and limitations can compromise the effectiveness of AI across different industries. For example, AI-driven HR (human resources) tools may unintentionally lead to discrimination against certain groups due to insufficient historical data of those demographics.
Recent studies have revealed substantial inaccuracies in the models used for determining loan approvals or rejections, particularly affecting minority populations. Research conducted by leading universities in the United States has demonstrated that the variable credit histories of different ethnicities often contribute to disparities in mortgage approval rates between majority and minority groups. It is imperative to conduct a thorough analytical review of the data; otherwise, the underlying causes of these biases may remain hidden and unaddressed. Additionally, in the healthcare sector, biased datasets can impact AI systems designed for diagnosing illnesses or recommending treatments. The consequences of such biases not only undermine trust in technology but also exacerbate existing inequalities, highlighting the critical need for inclusivity and meticulous oversight in the development of AI.
Large language models (LLMs), such as ChatGPT, are designed to generate content in response to human inquiries. Classified as generative AI, they excel at generating efficient responses to user prompts. However, this type of AI is not without its limitations, particularly in terms of issues related to bias and discrimination. Research indicates that LLMs demonstrate significant biases against women and individuals of Black ethnicity (Figure 2).
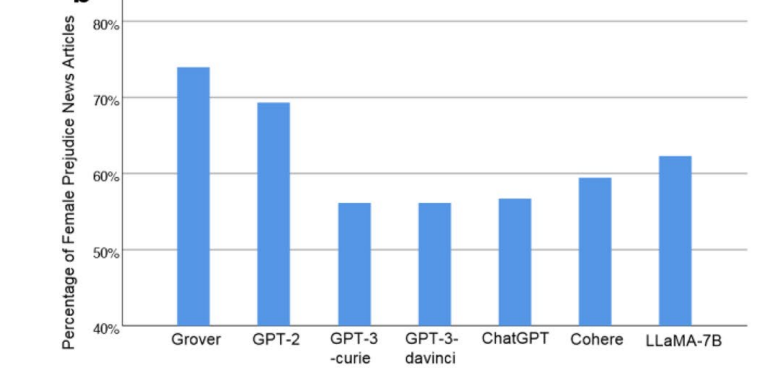
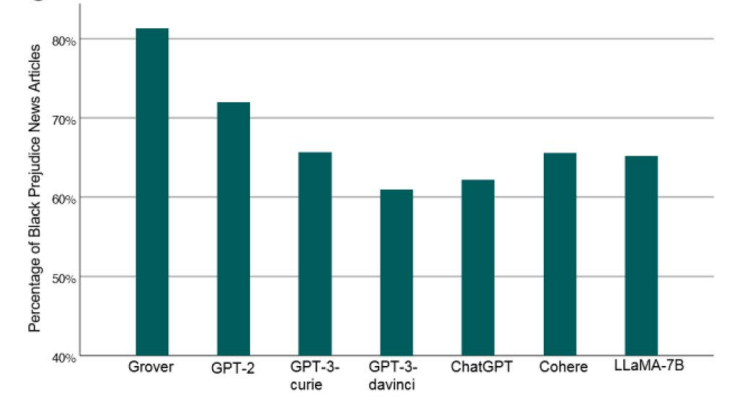
In a thorough examination of 555 AI models, researchers found that a staggering 83.1%, or 461 models, displayed a significant risk of bias (ROB). Furthermore, 71.7%, amounting to 398 models, suffered from insufficient sample sizes. Alarmingly, an overwhelming 99.1% of 550 models demonstrated inadequate handling of data complexity.
To truly harness the vast possibilities that AI presents, it is essential to develop and deploy these technologies thoughtfully and securely, with a unified effort to expand their benefits across society. In addition to biases in algorithms, AI may also cause privacy encroachments, harassment, the dissemination of fake news, and socio-economic ramifications, such as potential job displacement. Acknowledging and tackling these complex issues will be vital in nurturing the responsible growth and application of AI, paving the way for its broader acceptance and integration in our future.
What are the different types of biases and their corresponding reasons?
It is essential to assess and analyze the various types of biases to effectively address relevant challenges. The outcomes of AI systems can be influenced by different forms of bias. One notable example is implicit bias, which refers to the unconscious discrimination or prejudice that individuals may hold against others. This type of bias can be particularly detrimental, as those who exhibit it are often unaware of its presence. Additionally, bias can arise from statistical errors, commonly referred to as sample bias. This occurs when there is an insufficient representation of the actual population, leading to skewed results. Temporal bias may also be present in the datasets utilized for an AI application if they fail to consider potential changes that occur beyond a specific timeframe. Furthermore, overfitting can occur when an AI model performs well on training datasets but loses accuracy when faced with new data. Finally, bias may manifest in the inability to adequately address outliers—data points that fall outside the normal distribution of the dataset.
When companies depend on external vendors for AI services, ensuring data confidentiality becomes a complex challenge. This is particularly the case as sensitive information is sourced from the digital landscape across various platforms located in different, sometimes undisclosed, countries. The intricate web of diverse regulations governing data storage, access, and distribution across various nations presents significant obstacles, complicating the pursuit of secure and compliant data management in an increasingly interconnected world.
Responsible AI: Policy and Practice
Eminent figures such as SpaceX CEO Elon Musk and Apple co-founder Steve Wozniak signed a petition in March 2023, advocating for a six-month pause in AI research and development to tackle issues related to regulation and ethics. Ironically, just a month later, Musk launched a new AI-powered chatbot. Consequently, the promise of responsible AI for the public good is increasingly overshadowed by economic ambitions, which shift technological innovation away from fostering social inclusion and toward a competitive landscape defined by the pursuit of technological supremacy.
AI has firmly transcended the realms of business and commerce, now deeply influencing various spheres of social, political, and national domains. It has emerged as a transformative force across diverse sectors, fundamentally reshaping economic landscapes, societal structures, and the nature of work. This evolution has a significant impact on policy-making. Governments are increasingly framing AI as a vital tool for reshaping national identities and enhancing competitive advantages in the global economy. At the same time, they are investigating methods to leverage AI for social welfare and public benefit. However, these initiatives necessitate that lawmakers proactively address the risks and challenges associated with AI.
The intersection of research, policy, and technological development necessitates a critical examination to ensure that AI serves to bridge disparities rather than exacerbate them. A salient example of this discourse is the pursuit of gender equity through the strategic deployment of AI technologies. While AI holds significant promise as a transformative force for advancing gender equity, its efficacy is contingent on the establishment of intentional and inclusive policy frameworks.
The UK government has recently released a research report outlining its commitment to ‘Assuring a Responsible Future for AI’. This comprehensive, research-informed policy roadmap provides valuable insights into forthcoming initiatives. The document identifies a range of priorities, including safety and equity, to ensure the responsible deployment of AI technologies. Additionally, it emphasizes the importance of addressing the AI ecosystem to create a co-creative and regulated environment that mitigates the risks associated with the unethical and unequal use of AI. Currently, there are approximately 524 businesses in the UK offering AI assurance products and services, including 84 dedicated AI assurance firms. Together, these 524 companies generate an estimated revenue of £1.01 billion and employ approximately 12,572 people, positioning the UK's AI assurance sector as larger in relation to its economic scale compared to those in the US, Germany, and France. Figure 3 provides a framework for UK government’s initiatives with AI assurance.
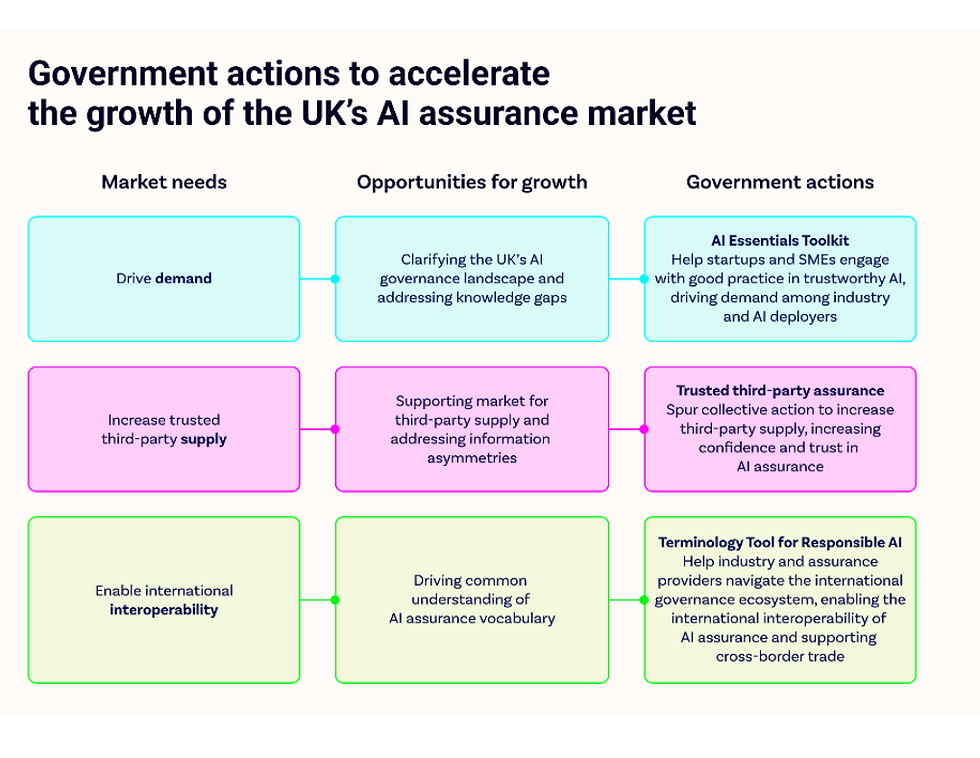
In 2024, EU member states unanimously passed the AI Act, similar to the General Data Protection Regulation (GDPR). This is deemed a significant milestone in regulating AI deployment. According to the act, any company using AI must comply with a wide range of issues, including people’s privacy and security. The act has received some criticism due to the operational challenges[1]. However, this is a move in a right direction, which will help to mitigate some of the inherent risks of AI.
The White House Blueprint for an AI Bill of Rights outlines five key principles and guidance for practice, intended to inform the design, implementation, and deployment of AI[2]. These principles focus on ensuring safety and effectiveness, preventing algorithmic bias and discrimination, safeguarding data privacy, providing clear notice and explanations, and ensuring the availability of human alternatives, considerations, and fallback options.
Policies and regulations aimed at ensuring the responsible deployment and use of AI are still in their formative stages. It is important to recognize that different countries may prioritize distinct contextual factors. AI-related policies also reflect a nation's and its government's perception of various challenges, as well as their national objectives in promoting cutting-edge technologies in preferred sectors. Nonetheless, these policies and regulations should be supportive rather than restrictive, as overly stringent measures may hinder the broader application of AI and its immense potential. It is also important to remember that this is an evolving landscape where innovation comes thick and fast., Therefore, alongside legal requirements and policy guidelines, it is essential to establish strong ethical codes that should guide business and management practices.
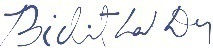
Dr Bidit L. Dey
Associate Professor in Marketing
Sheffield University Management School (the University of Sheffield), UK
LinkedIn: Bidit Dey | LinkedIn
Additional references: